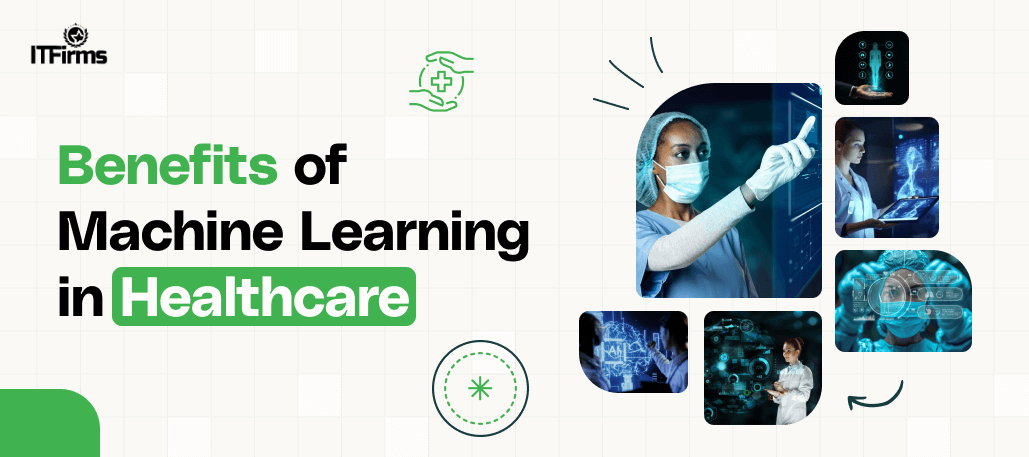
Benefits of Machine Learning in Healthcare!
Machine Learning in Healthcare is promising for numerous scalable benefits; automation, effective treatment plans, and better patient care. Read and acknowledge the worth of ML in Healthcare.
The healthcare industry is continuously experimenting with new technologies and upgrading with advancements and automation task processes. Along with the tech experimenting, Machine Learning in Healthcare came out with a big change. It enables better patient care, produces deep medical insights, and helps healthcare professionals with treatment.
“Do You Know: 86% of healthcare organizations are using ML in some form, and more than 80% of the organizations are prepared with robust AI integration plans. ML has become a significant disciplinary protocol larger than AI expansions.”
Many SMEs and Large healthcare organizations plan to integrate Machine Learning into their systems. There are many perks associated with it. Here this blog will elaborate on the benefits of machine learning in the healthcare industry.
Consider these advantages, and acknowledge their impact on your healthcare organization to gain worth into your operations.
What is Machine Learning?
Machine Learning is another advanced form of Artificial Intelligence, which studies huge data and identifies the patterns to bring prompt result-oriented outcomes. Instead of commanding computers for everything, Machine Learning (ML) observes data and analyzes the patterns to find the right solutions based on the requirements.
In the healthcare industry, Machine Learning (ML) helps in delivering personalized treatment, AI-assisted surgeries, earlier disease detection, medical imaging, fraud identification, prescription analysis, etc.
With the advanced perks of Machine Learning in healthcare, organizations are growing fast as well as bringing precision in the medicinal industry. The patients are potent in gaining efficient treatments and professionals are capable of retrieving important information about diseases, medicines, and patient’s conditions.
Benefits of Machine Learning in Healthcare
The machine learning algorithm is a valuable asset to the healthcare industry. It has access to the humongous database assembled in the electronic health records (EHR) and retrieves the required insights immediately. Besides being good at databases, ML is also great with other various operational things. So here are some benefits of ML in Healthcare listed:
Automation with the Routine Tasks
Machine Learning enables managing the EHR systems and helps in virtual nursing as well. It improves efficiency and automates various tasks with accuracy. ML studies vast amounts of data, identifies the patterns, aids in early diagnosis, and lastly prepares personalized treatment plans for the patients. However, it also does automated AI image analysis in radiology, detects anomalies like tumors with precision, etc.
Moreover, the ML algorithms also streamline the administrative regular tasks; such as patient record management, fixing the schedules, reducing the healthcare staff’s hefty workloads, and more of that. Additionally, the empowerment of predictive analysis associated with ML enables optimizing resource allocation and maintaining the patient care workflows leading to better patient care and efficient treatment.
Improved Decision-Making Power
Machine Learning is known to deal with huge databases and analyze their patterns to identify the right solutions. It detects the enormous datasets, in which healthcare professionals can streamline their diagnostics and choose better decision-making.
With the studies conducted on healthcare datasets, the tech-enabled tools and software suggest the best advice for the best patient healthcare and their treatments. Through better bits of advice, healthcare professionals can bring better patient condition outcomes. Also, the advice suggested by the ML’s evaluation will help professionals gain better insights and improve their decision-making power. This is how the treatments get better and patients get more personalized treatments.
Accelerated Drug Discoveries and Innovation
Without ignoring the fact that, Machine Learning is the subfield of AI which studies datasets across the globe. The technology has retained huge information from healthcare, which will help researchers figure out the best options during drug discoveries.
Integrating machine learning with deep learning enables the discovery of drugs and can create innovations in the medicinal industry. The researchers can build more accurate models; which have the potential to successfully study and predict the drug molecules. It enables quickening up the drug discovery processes and finding new drugs in the medicinal industry to cure more of some anonymous health issues.
Quick Data Collection
Well, the concept of machine learning is very clear to conduct deep analysis on huge datasets. However, healthcare professionals are using wearable technology to compile real-time data, whereas Machine Learning can process that very quickly. This is why the U.S. Food and Drug Administration has been working to integrate AI with ML in creating some medical software to study data quickly and show efficient results.
In case you need to shortlist the data, there’s no need for hours of wasting manual efforts with ML. The machine learning algorithms can process real-time existing data in minutes and shortlist the required data easily to finish work immediately.
Enhanced Patient Experiences
Through ML, healthcare organizations integrate virtual assistants and chatbots into their systems. Whenever the patients are in doubt about anything, they can use and ask queries through the chatbots. The advanced technology reads human behavior and checks its requirements deeply. The huge datasets gathered in the ML-integrated systems will study queries and share solutions based on whatever has been asked through NLP. It enhances the patient’s experience with the healthcare organizations and also builds loyalty among them. On the other hand, healthcare professionals can also get help from ML and retrieve deep insights into patients’ health conditions to suggest more personalized treatment. This is how machine learning in healthcare is helping to improve the overall experience of the patients.
Extended Access to Healthcare Parameters
There will be no compromises with the healthcare treatments of patients when the systems are integrated with machine learning. With the help of vast amounts of data, healthcare professionals have deep access to any health problem, which enables them to make the right personalized decision on the patient’s treatment. The extended approach to the healthcare parameters can help the professional to gain better insights and patients receive treatment that is accurate based on their condition.
Reduced Risks with AI/ML
No denying that AI/ML in sports can access huge data, it can identify patterns, learn about any possible mishaps, make suggestions based on historical information, etc. ML helps figure out how to make the game better, how the athletes can perform better, and more to help the fans stay engaged. Alongside making things accurate, it also prevents the wrong things from happening with the help of risk assessment. The adoption of ML in sports allows early detection of serious problems and injuries, which helps reduce the risks further.
Most importantly, this advanced tech-enabled algorithm identifies possible injuries that happen to athletes. With the help of early injury detection, it shares the preventive measures to protect from high risks and possible surgeries to players. However, these preventions keep athletes more health conscious and fit to play better in the fields.
Cost-Effective with ML in Healthcare
There are plenty of works that can be done with the help of machine learning in healthcare systems. However, it also assists in doing regular tasks with automations. These algorithms can help in numerous tasks; such as quickly scanning the EHRs for certain patient data, scheduling the appointment with patients, automating some medical procedures, etc. Fortunately, the advancement of technology has helped a lot in general tasks in healthcare organizations, which is why professionals can focus on other serious matters.
Use-Cases of Machine Learning in the Healthcare Industry
Machine Learning in Healthcare is useful for several purposes. Listed are some of those use cases of Machine Learning in Healthcare Industry:
Patient Behavior Modification
Several diseases can be avoidable if detected at its earliest stage; such as obesity, diabetes, heart diseases, and many more. Here machine learning helps by retrieving the health data of patients from their connected medical devices and sensors. With the earlier detection of any disease, patients can control their condition and become better.
Virtual Nursing
Healthcare organizations are using virtual nursing through machine learning, which manages doctors’ appointments, schedules their meet-ups, calls in emergencies or any major health alerts, manages post-discharge care, and analyzes patients’ conditions now and then. These virtual nurses are computer-generated avatars, which are designed to be available for patients 24/7 and respond immediately to patient’s any need.
Medical Imaging
The analysis of medical images was prone to human error back in time. Manually, the professionals might leave some important things unseen. With the help of machine learning, we can scan medical images without leaving any part of it. Moreover, this can identify the subtlest changes in CT scans, X-rays, MRIs, etc. Radiologists take help from ML to detect the images and diagnose diseases.
Hospital Management Optimization
The surging demands for healthcare services are increasing day by day, which is why hospitals and other big healthcare organizations need a streamlined management system. With the ML-based systems, you can master administrative data processing and also facilitate the hospitals to execute various functions; such as staff-scheduling, supply chain, resource allocation, inventory management, compliance monitoring, and more.
Robot-Assisted Surgery
Well, using robot assistance during surgeries has been in trend for years. Through the ML-integrated robots; it increases the accuracy during surgeries and reduces the duration of operations. The ML-enabled robots can perform complicated surgeries, minimize the patient’s trauma, and lessen the recovery time. The robots are fed with required data, which helps healthcare professionals and doctors to perform surgeries with more precision.
Accurate Diagnosis and Higher-Risk Identification
An inaccurate diagnosis of a patient’s health can detriment their condition more badly. Therefore, machine learning has received better reviews from healthcare organizations to help them with more accuracy and determination on patient’s healthcare diagnoses and treatments. Combining machine learning with current systems enables the identification of patterns and processes with automation to provide streamlined diagnosis and treatment.
What is the Difference Between Machine Learning and Deep Learning in Healthcare?
The healthcare industry is taking leverage from Machine Learning (ML) as well as Deep Learning (DL). Both have their perks, which enable organizations and professionals to enhance diagnostics, treatment, and patient care.
Machine Learning:
It involves algorithms that learn from structured data. This means human experts need to pre-process the data and select the features that the algorithm will analyze. For instance, in predicting disease outcomes, features like patient age, symptoms, and medical history are manually inputted. ML models, such as decision trees and support vector machines, then use these features to make predictions. ML is highly effective when dealing with smaller datasets and problems where the features are well understood.
Deep Learning:
It is a subset of ML, utilizing neural networks with many layers (hence “deep”) to automatically discover patterns in data. This capability makes DL particularly powerful for analyzing unstructured data, like medical images, genomic sequences, and natural language from clinical notes. For example, DL models can be trained to recognize anomalies in X-rays or MRI scans, often surpassing human accuracy. DL requires large datasets and significant computational power but excels in tasks where the features are not easily defined by humans.
ML is best for applications with structured data and clearly defined features, whereas DL is suitable for complex, high-dimensional data requiring automated feature extraction. Both technologies are advancing healthcare by improving diagnostic accuracy, personalizing treatments, and enabling predictive analytics.
Aspect | Machine Learning | Deep Learning |
Data Type | Only Structured Data | Structured and Unstructured Data |
Feature Extraction | Manual | Automatic |
Algorithm Complexity | Simpler Algorithm (Decision Trees) | Complex Algorithm (Deep Neural Networks) |
Data Requirement | Small Datasets | Large Database |
Computational Power | Moderate | High |
Use-Cases | Predicting Disease Outcomes, and Patient Segmentation | Medical Imaging, Genomics, Natural Language Processing (NLP) |
By understanding these differences, healthcare professionals can better leverage ML and DL technologies to improve patient outcomes and operational efficiencies.
Applications of Machine Learning in Healthcare
Numerous healthcare companies are using machine learning to improve database accessibility, improve patient’s health, and enhance diagnostic analysis:
The Viz.AI is another machine learning tool, which is used to optimize care coordination and improve communication between healthcare professionals. The software also helps streamline care by connecting the healthcare providers to experts about the patient’s condition, which results in quick improvement in the patient’s health.
Pfizer is using IBM’s Watson AI technology and Natural Language Processing (NLP) for immuno-oncology research, where it’s been analyzed how the immune systems can fight against cancer. Also, the collaboration of Pfizer with machine learning has helped in doing deep analysis of huge patient data and retrieving significant insights from that to build more accurate immuno-oncology treatments for the patients based on their condition.
Deep Genomics is another AI-powered platform, which helps in conducting deep research by assisting healthcare professionals to quickly find resources for drug development to cure specific diseases and medical problems. The implementation of ML helps in identifying the potential reasons causing genetic health issues. The system learns about how the person’s mutation changes an individual’s DNA and causes medical concerns. Also, machine learning solutions can figure out possible medicines and resolutions for certain particular diseases.
Insitro uses machine learning and computational biology to make drug development faster and more affordable. By creating predictive models from vast amounts of biological data, Insitro can identify important trends, such as new disease subtypes. This allows health professionals to fine-tune drugs and treatments, offering better protection for patients against emerging diseases.
Intuitive Surgical is behind the Da Vinci Surgical System, the most widely used machine learning-powered surgical system. The Da Vinci system enables surgeons to perform minimally invasive, robotic-assisted surgeries, which greatly improve patient outcomes and reduce recovery times.
PathAI leverages machine learning to assist pathologists in making more accurate diagnostic decisions. The company creates algorithms for clinical trials and develops ML models to optimize the analysis of patient tissue samples. By collaborating with leading drug developers and healthcare organizations, PathAI is expanding the role of artificial intelligence in healthcare diagnostics.
Oncora Medical
Oncora Medical, a Philadelphia-based startup, is transforming cancer research and treatment by collecting extensive data from medical records, EHRs, cancer registries, and other systems. Oncora’s platform evaluates care quality and suggests improved treatment methods. The company treats the development of machine learning models with the same rigor as biopharmaceutical companies, ensuring each model undergoes a comprehensive, multi-phase assessment process.
Final Verdict
Machine Learning (ML) is the advancement of AI, which has helped healthcare organizations to immersively bring more accuracy in diagnostics, treatment, and patient healthcare. The enormous database ML consists in itself can get done with things so easily and even potent to automate several regular tasks though.
Machine Learning (ML) has a promising future afterward, which is going to prevent drastic outbreaks across the globe. This advanced technology can analyze huge databases, provide personalized healthcare treatment, predict risks, perform various tasks, and do a lot.
Lastly, Healthcare Machine Learning solutions have a lot of advantages to offer for healthcare professionals and patients. This advancement is only rising, and reaching more precision. Acknowledge the technology and bring the best out of it for yourself indeed.
Frequently Asked Questions (FAQs)
How is Machine Learning Used in Healthcare?
Healthcare organizations can use machine learning in several ways; such as helping with disease diagnosis, treatment planning, drug discovery, predicting outcomes, effective patient care, efficient at detecting anomalies, etc. Moreover, the advanced technology can identify anonymous patterns, receive deep insights into the patient’s condition, and result in suggesting better-personalized treatment plans to deliver the best for the betterment of patients. Additionally, healthcare organizations have also streamlined other administrative operations and automated various regular tasks.
What’s the Future Scope of Machine Learning in Healthcare?
Machine learning in Healthcare is completely transitioning the industry. With the surge of AI in Healthcare, ML introduced easier accessibility with a huge database, revolutionized the way healthcare professionals conduct research, perform operational tasks, and treat patients, resulting in efficiency with the patient’s health outcomes, and also increased productivity in the healthcare organization with automation. The advanced technology “Machine Learning” in the Healthcare Industry has a futuristic scope with more enriched and promising features.
How Does ML Reduce Cost in the Healthcare Industry?
No denying that integrating machine learning technology into the existing healthcare system is going to be costlier, but eventually that becomes an affordable option due to the reduced cost in other sections. For instance; there are lots of tasks that can be automated and done repeatedly with machine learning, which results in saving costs at manual hiring. There are many other ways ML is reducing the cost of Healthcare organizational operations. So the emergence brought by machine learning development companies is helping to save huge costs.
Who Can Use Machine Learning in Healthcare?
Not just Healthcare organizations; but pharmaceutical companies, healthcare providers, technology companies, government institutes, etc can process machine learning app development to benefit them with numerous perks like automation, better patient treatment, deep insights on patient health measures, etc.